2020. 3. 3. 07:21ㆍ카테고리 없음
In, the Breusch–Godfrey test, named after and, is used to assess the validity of some of the modelling assumptions inherent in applying models to observed data series. In particular, it for the presence of that has not been included in a proposed model structure and which, if present, would mean that incorrect conclusions would be drawn from other tests, or that sub-optimal estimates of model parameters are obtained if it is not taken into account; the regression models to which the test can be applied include cases where lagged values of the are used as in the model's representation for later observations.
This type of structure is common in.Because the test is based on the idea of, it is sometimes referred to as LM test for serial correlation.A similar assessment can be also carried out with the and the. Breusch, T. 'Testing for Autocorrelation in Dynamic Linear Models'. Australian Economic Papers. 17: 334–355. Godfrey, L.
'Testing Against General Autoregressive and Moving Average Error Models when the Regressors Include Lagged Dependent Variables'. 46: 1293–1301. Asteriou, Dimitrios; Hall, Stephen G. Applied Econometrics (Second ed.).
New York: Palgrave Macmillan. Pp. 159–61. CRAN. Kleiber, Christian; Zeileis, Achim (2008). Applied Econometrics with R. New York: Springer.
Pp. 104–106. (PDF). Stata Manual. Baum, Christopher F.
An Introduction to Modern Econometrics Using Stata. Pp. 155–158. Breusch-Godfrey test in Python 2014-02-28 at theFurther reading. Godfrey, L.
Misspecification Tests in Econometrics. Cambridge, UK: Cambridge. Godfrey, L. 'Misspecification Tests and Their Uses in Econometrics'. Journal of Statistical Planning and Inference. 49 (2): 241–260.; Lahiri, Kajal (2009).
Introduction to Econometrics (Fourth ed.). Chichester: Wiley. In, the harmonic mean is one of several kinds of average, in particular one of the Pythagorean means. It is appropriate for situations when the average of rates is desired; the harmonic mean can be expressed as the reciprocal of the arithmetic mean of the of the given set of observations. As a simple example, the harmonic mean of 1, 4, 4 is − 1 = 3 1 1 + 1 4 + 1 4 = 3 1.5 = 2. The harmonic mean H of the positive real numbers x 1, x 2, x n is defined to be H = n 1 x 1 + 1 x 2 + ⋯ + 1 x n = n ∑ i = 1 n 1 x i = − 1; the third formula in the above equation expresses the harmonic mean as the reciprocal of the arithmetic mean of the reciprocals.
From the following formula: H = n ⋅ ∏ j = 1 n x j ∑ i = 1 n. It is more apparent that the harmonic mean is related to the arithmetic and geometric means.
It is the reciprocal dual of the arithmetic mean for positive inputs: 1 / H = A The harmonic mean is a Schur-concave function, dominated by the minimum of its arguments, in the sense that for any positive set of arguments, min ≤ H ≤ n min.Thus, the harmonic mean cannot be made arbitrarily large by changing some values to bigger ones. The harmonic mean is one of the three Pythagorean means. For all positive data sets containing at least one pair of nonequal values, the harmonic mean is always the least of the three means, while the arithmetic mean is always the greatest of the three and the geometric mean is always in between, it is the special case M−1 of the power mean: H = M − 1 = n x 1 − 1 + x 2 − 1 + ⋯ + x n − 1 Since the harmonic mean of a list of numbers tends toward the least elem. Gangadharrao Soundalyarao 'G. Maddala was an economist and teacher, known for his contributions in the field of and for the textbooks he authored in this field. He was 'born in to a family of modest means.'
He obtained a B. In from and, in 1957, an M. In Statistics from. He came to the as a to the Economics department of the.
In 1963 he completed his Ph. In the Department of Economics at the University of Chicago with a dissertation written under the supervision of. Maddala's first faculty position was at, he held the University Eminent Scholar position at upon his death. Maddala published over 110 scholarly papers and wrote 12 books covering most of the emerging areas of econometrics, his 1983 book titled Limited Dependent and Qualitative Variables in Econometrics is now regarded as a classic and seminal text for advanced studies in econometrics.In econometrics methodology, Maddala’s key areas of research and exposition included distributed lags, panel data, simultaneous equations, errors in variables, income distribution, switching regressions, disequilibrium models and models, models and methods, unit roots and methods, Bayesian econometrics. In empirical economics, Maddala contributed to the areas of consumption and cost functions, money demand, pseudo-data, returns to college education, housing market discrimination, survey data on expectations, risk premia in future markets. ' in the Industry, 1919-1954'.
Dissertation, The University of Chicago, United States—Illinois. Limited Dependent and Qualitative Variables in Cambridge. Description, preview.
Introduction to Econometrics, 2nd ed. Macmillan; the Memorial Fund, at Ohio State University, was created in 2004, by Dr. Maddala's wife and colleagues and students.It provides awards 'to graduate students for excellence in quantitative research using econometrics, both theoretical and applied' and sponsors distinguished speakers. Maddala, 'How I Became an Econometrician', autobiographic essay Kajal Lahiri and, 'Obituary: G. Maddala, 1939-1999', 15 Kajal Lahiri, 'The ET Interview: Professor G.
Serial Correlation Test In R

Maddala', Econometric Theory 15. Statistics is a branch of dealing with data collection, analysis and presentation. In applying statistics to, for example, a scientific, industrial, or social problem, it is conventional to begin with a statistical population or a statistical model process to be studied.
Populations can be diverse topics such as 'all people living in a country' or 'every composing a crystal'. Statistics deals with every aspect of data, including the planning of data collection in terms of the design of surveys and experiments. See; when census data cannot be collected, statisticians collect data by developing specific experiment designs and survey samples. Representative sampling assures that inferences and conclusions can reasonably extend from the sample to the population as a whole. An experimental study involves taking measurements of the system under study, manipulating the system, taking additional measurements using the same procedure to determine if the manipulation has modified the values of the measurements.In contrast, an observational study does not involve experimental manipulation.
Two main statistical methods are used in data analysis: descriptive statistics, which summarize data from a sample using indexes such as the mean or standard deviation, inferential statistics, which draw conclusions from data that are subject to random variation. Descriptive statistics are most concerned with two sets of properties of a distribution: central tendency seeks to characterize the distribution's central or typical value, while dispersion characterizes the extent to which members of the distribution depart from its center and each other. Inferences on mathematical statistics are made under the framework of probability theory, which deals with the analysis of random phenomena.
A standard statistical procedure involves the test of the relationship between two statistical data sets, or a data set and synthetic data drawn from an idealized model. A hypothesis is proposed for the statistical relationship between the two data sets, this is compared as an alternative to an idealized null hypothesis of no relationship between two data sets.Rejecting or disproving the null hypothesis is done using statistical tests that quantify the sense in which the null can be proven false, given the data that are used in the test. Working from a null hypothesis, two basic forms of error are recognized: Type I errors and Type II errors. Multiple problems have come to be associated with this framework: ranging from obtaining a sufficient sample size to specifying an adequate null hypothesis. Measurement processes that generate statistical data are subject to error. Many of these errors are classified as random or systematic, but other types of errors can be important; the presence of missing data or censoring may result in biased estimates and specific techniques have been developed to address these problems. Statistics can be said to have begun in ancient civilization, going back at least to the 5th century BC, but it was not until the 18th century that it started to draw more from and probability theory.
In more recent years statistics has relied more on statistical software to produce tests such as descriptive analysis.Some definitions are: dictionary defines statistics as 'a branch of mathematics dealing with the collection, analysis and presentation of masses of numerical data.' Defines statistics as 'Numerical statements of facts in any department of inquiry placed in relation to each other.' Statistics is a mathematical body of science that pertains to the collection, interpretation or explanation, presentation of data, or as a branch of mathematics.
Some consider statistics to be a distinct mathematical science rather than a branch of mathematics. While many scientific investigations make use of data, statistics is concerned with the use of data in the context of uncertainty and decision making in the face of uncertainty. Mathematical statistics is the application of to statistics. Mathematical techniques used for this include mathematical analysis, linear, stochastic analysis, differential equations, probability theory.In applying statistics to a problem, it is common practice to start with a population or process to be studied. Populations can be diverse topics such as 'all people living in a country' or 'every composing a crystal'. Ideally, statisticians compile data about the entire population; this may be organized by governmental statistical institutes. Descriptive statistics can be used to summarize the population data.
Numerical descriptors include mean and standard deviation for continuous data types, while frequency and percentage are more useful in terms of describing categorical data; when a census is not feasible, a chosen subset of the population called. Once a sample, representative of the population is determined, data is collected for the sample members in an observational or experimental setting. Again, descriptive statistics can be used to summarize the sample data. However, the drawing of the sample has been subject to an element of randomness, hence the established numerical descriptors from the sample are due to uncertainty.To still draw meaningful conclusions about the entire population, in. In statistics, linear regression is a linear approach to modelling the relationship between a response and one or more explanatory variables. The case of one explanatory variable is called. For more than one explanatory variable, the process is called multiple linear regression; this term is distinct from multivariate linear regression, where multiple correlated dependent variables are predicted, rather than a single scalar variable.
In linear regression, the relationships are modeled using linear predictor functions whose unknown model parameters are estimated from the data; such models are called linear models. Most the of the response given the values of the explanatory variables is assumed to be an of those values.
Like all forms of regression analysis, linear regression focuses on the of the response given the values of the predictors, rather than on the joint probability distribution of all of these variables, the domain of multivariate analysis.Linear regression was the first type of regression analysis to be studied rigorously, to be used extensively in practical applications. This is because models which depend linearly on their unknown parameters are easier to fit than models which are non-linearly related to their parameters and because the statistical properties of the resulting estimators are easier to determine. Linear regression has many practical uses. Most applications fall into one of the following two broad categories: If the goal is prediction, or forecasting, or error reduction, linear regression can be used to fit a predictive model to an observed data set of values of the response and explanatory variables. After developing such a model, if additional values of the explanatory variables are collected without an accompanying response value, the fitted model can be used to make a prediction of the response. In statistics, is a type of linear least squares method for estimating the unknown parameters in a linear regression model.
OLS chooses the parameters of a linear function of a set of explanatory variables by the principle of least squares: minimizing the sum of the squares of the differences between the observed dependent variable in the given dataset and those predicted by the linear function. Geometrically, this is seen as the sum of the squared distances, parallel to the axis of the dependent variable, between each data point in the set and the corresponding point on the regression surface – the smaller the differences, the better the model fits the data; the resulting estimator can be expressed by a simple formula in the case of a, in which there is a single regressor on the right side of the regression equation. The OLS estimator is consistent when the regressors are, optimal in the class of linear unbiased estimators when the errors are and serially.Under these conditions, the method of OLS provides minimum-variance mean-unbiased estimation when the errors have finite variances.
Under the additional assumption that the errors are distributed, OLS is the maximum likelihood estimator. OLS is used in fields as diverse as economics, data science, political science and engineering. Suppose the data consists of n observations ni=1; each observation i includes a response yi and a column vector xi of values of p predictors xij for j = 1. In inferential statistics, the null hypothesis is a general statement or default position that there is no relationship between two measured phenomena, or no association among groups. Testing the null hypothesis—and thus concluding that there are or are not grounds for believing that there is a relationship between two phenomena —is a central task in the modern practice of science; the null hypothesis is assumed to be true until evidence indicates otherwise. In statistics, it is denoted H0; the concept of a null hypothesis is used differently in two approaches to statistical inference. In the significance testing approach of, a null hypothesis is rejected if the observed data are unlikely to have occurred if the null hypothesis were true.
In this case the null hypothesis is rejected and an alternative hypothesis is accepted in its place. If the data are consistent with the null hypothesis the null hypothesis is not rejected. In neither case is the null hypothesis or its alternative proven.This is analogous to the legal principle of, in which a suspect or defendant is assumed to be. In the hypothesis testing approach of and, a null hypothesis is contrasted with an alternative hypothesis and the two hypotheses are distinguished on the basis of data, with certain error rates, it is used in formulating answers in researches.
Statistical inference can be done without a null hypothesis, by specifying a statistical model corresponding to each candidate hypothesis and using model selection techniques to choose the most appropriate model. Hypothesis testing requires constructing a statistical model of what the data would look like, given that chance or random processes alone were responsible for the results; the hypothesis that chance alone is responsible. The model of the result of the random process is called the distribution under the null hypothesis; the obtained results are compared with the distribution under the null hypothesis, the likelihood of finding the obtained results is thereby determined.Hypothesis testing works by collecting data and measuring how the particular set of data is, assuming the null hypothesis is true, when the study is on a randomly selected representative sample. The null hypothesis assumes no relationship between variables in the population from which the sample is selected.

If the data-set of a randomly selected representative sample is unlikely relative to the null hypothesis, the experimenter rejects the null hypothesis concluding it is false; this class of data-sets is specified via a test statistic, designed to measure the extent of apparent departure from the null hypothesis. The procedure works by assessing whether the observed departure measured by the test statistic is larger than a value defined so that the probability of occurrence of a more extreme value is small under the null hypothesis. If the data do not contradict the null hypothesis only a weak conclusion can be made: namely, that the observed data set provides no strong evidence against the null hypothesis.In this case, because the null hypothesis could be true or false, in some contexts this is interpreted as meaning that the data give insufficient evidence to make any conclusion. For instance, a certain drug may reduce the chance of having a heart attack. Possible null hypotheses are 'this drug does not reduce the chances of having a heart attack' or 'this drug has no effect on the chances of having a heart attack'; the test of the hypothesis consists of administering the drug to half of the people in a study group as a controlled experiment.
If the data show a statistically significant change in the people receiving the drug, the null hypothesis is rejected; the null hypothesis and the alternative hypothesis are types of conjectures used in statistical tests, which are formal methods of reaching conclusions or making decisions on the basis of data. The hypotheses are conjectures about a statistical model of the population, which are based on a sample of the population; the tests are core elements of statistical inference used in the interpretation of scientific experimental data, to separate scientific claims from statistical noise.' The statement being tested in a test of statistical significance is called the null hypothesis. The test of significance is designed to assess the strength of the evidence against the null hypothesis; the null hypothesis is a statement of'no effect' or'no difference'.'
It is symbolized as H0. The statement, being tested against the null hypothesis is the alternative hypothesis. Symbols include Ha.
Statistical significance test: 'Very the procedure f. The median is the value separating the higher half from the lower half of a data sample. For a data set, it may be thought of as the 'middle' value. For example, in the data set, the median is 6, the fourth largest, the fifth smallest, number in the sample. For a continuous probability distribution, the median is the value such that a number is likely to fall above or below it; the median is a used measure of the properties of a data set in statistics and probability theory. The basic advantage of the median in describing data compared to the mean is that it is not skewed so much by large or small values, so it may give a better idea of a 'typical' value. For example, in understanding statistics like household income or assets which vary a mean may be skewed by a small number of high or low values.
Median income, for example, may be a better way to suggest; because of this, the median is of central importance in robust statistics, as it is the most resistant statistic, having a breakdown point of 50%: so long as no more than half the data are contaminated, the median will not give an arbitrarily large or small result.The median of a finite list of numbers can be found by arranging all the numbers from smallest to greatest. If there is an odd number of numbers, the middle one is picked. For example, consider the list of numbers 1, 3, 3, 6, 7, 8, 9This list contains seven numbers; the median is the fourth of them, 6.
If there is an number of observations there is no single middle value. For example, in the data set 1, 2, 3, 4, 5, 6, 8, 9the median is the mean of the middle two numbers: this is / 2, 4.5. The formula used to find the index of the middle number of a data set of n numerically ordered numbers is / 2; this either gives the halfway point between the two middle values. For example, with 14 values, the formula will give an index of 7.5, the median will be taken by averaging the seventh and eighth values. So the median can be represented by the following formula: m e d i a n = a ⌈ # x ÷ 2 ⌉ + a ⌈ # x ÷ 2 + 1 ⌉ 2 One can find the median using the.
There is no accepted standard notation for the median, but some authors represent the median of a variable x either as x͂ or as μ1/2 sometimes M.In any of these cases, the use of these or other symbols for the median needs to be explicitly defined when they are introduced. The median is used for skewed distributions, which it summarizes differently from the arithmetic mean. Consider the; the median is 2 in this case, it might be seen as a better indication of central tendency than the arithmetic mean of 4.
The median is a popular summary statistic used in descriptive statistics, since it is simple to understand and easy to calculate, while giving a measure, more robust in the presence of values than is the mean; the cited empirical relationship between the relative locations of the mean and the median for skewed distributions is, not true. There are, various relationships for the absolute difference between them. With an number of observations no value need be at the value of the median. Nonetheless, the value of the median is uniquely determined with the usual definition. A related concept, in which the outcome is forced to correspond to a member of the sample, is the.In a population, at most half have values less than the median and at most half have values greater than it.
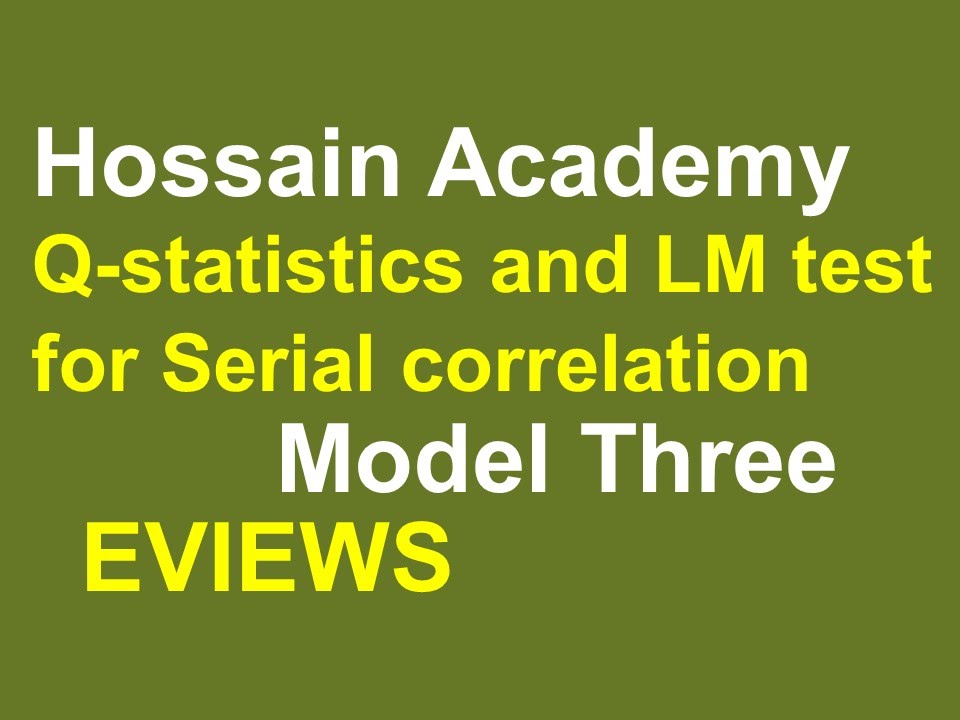
If each group contains less than half the population some of the population is equal to the median. For example, if a. 1875 illustration of the correlation between the heights of adults and their parents. The observation that adult children's heights tended to deviate less from the mean height than their parents suggested the concept of ', giving regression its name. The 'locus of horizontal tangential points' passing through the leftmost and rightmost points on the ellipse (which is a of the estimated from the data) is the estimate of the regression of parents' heights on children's heights, while the 'locus of vertical tangential points' is the OLS estimate of the regression of children's heights on parent's heights. The major axis of the ellipse is the estimate.